The world of locally deploying large language models is exploding with tutorials lately. Today, I’m excited to share my hands-on experience running massive AI models on an RTX 5090 setup – the good, the bad, and the surprising realities.
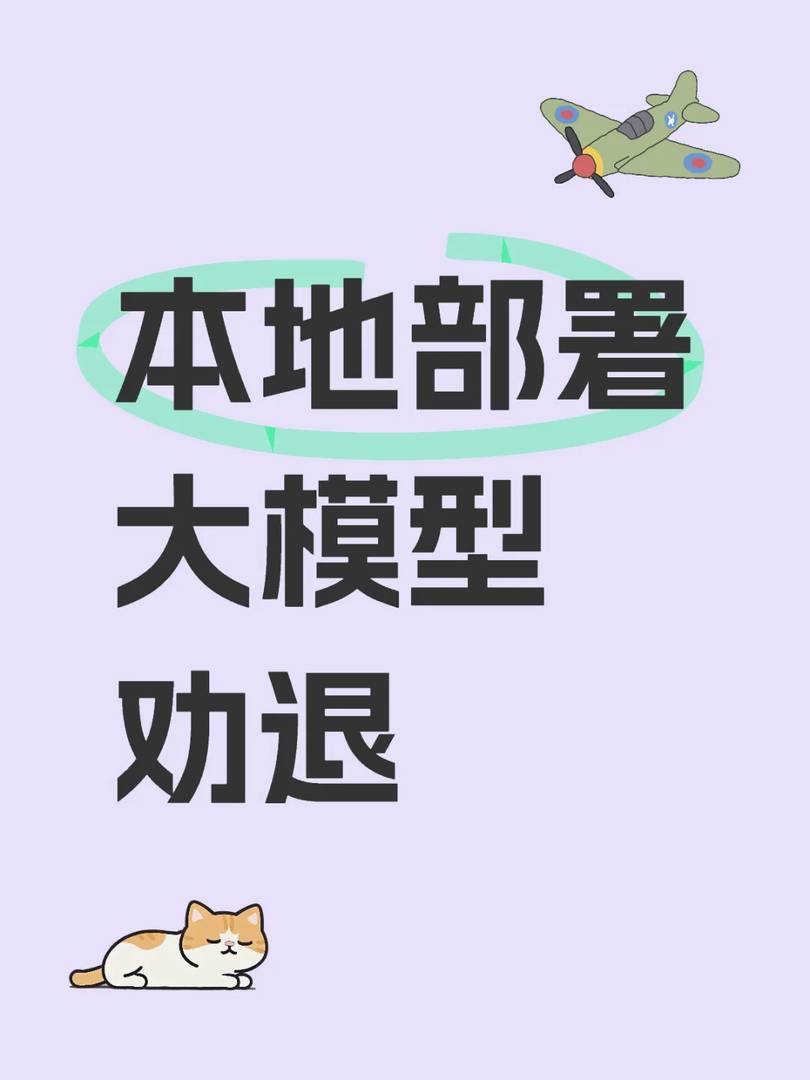
**My Powerhouse Setup:**
– GPU: Beastly RTX 5090 (32GB VRAM)
– CPU: Flagship i9-14900K
– RAM: 64GB of blazing-fast memory
– Tested Models: 32B Q4 quantized versions of qwq and deepseek r1 distilled
**Eye-Opening Discoveries:**
1. **Performance Insights:**
– The 32B Q4 model purrs along beautifully, churning out dozens of tokens per second like a well-oiled machine.
– But push it to 70B or 32B Q8, and you’ll hit VRAM’s unforgiving wall.
– Shared memory becomes a performance killer – we’re talking snail’s pace territory here.
2. **Brainpower Test (math & physics challenges):**
– The r1 32B shows promise on basic queries, like a bright student acing pop quizzes.
– Complex reasoning? That’s where it starts sweating bullets.
– The qwq 32B? Let’s just say it’s the class clown – often hilariously off the mark.
**The Hard Truths:**
1. Yes, your gaming GPU can moonlight as an AI workstation… for smaller models.
2. But commercial solutions? They’re in a different league entirely.
3. Right now, your wallet might cry more than your GPU does.
**Straight-Shooting Advice:**
– Perfect for weekend tinkerers and AI enthusiasts
– Temper those performance expectations – this isn’t ChatGPT-4
– Hold off on that hardware splurge (your bank account will thank you)
If you’re hungry for more, I’ll gladly serve up screenshots – just say the word! Hope this gives fellow LLM explorers a reality check before diving in. Drop your thoughts below – let’s geek out together!
— PC
That RTX 5090 setup sounds insane, but I’m not surprised it struggled with some of those bigger models. It’s wild how even top-tier hardware can still hit limits, especially when quantization doesn’t fully close the gap. I’d love to know more about how the different model versions compared in terms of performance tradeoffs!
It’s fascinating how much difference there is between different quantized model versions, especially since I didn’t realize the deepseek R1 distilled performed so well on your setup. I wonder if others with slightly lower-end GPUs could still get decent results using these lighter models?
Absolutely! Lighter models like DeepSeek R1 Distilled are designed to perform well even on modest hardware. Many users with mid-range GPUs have reported great results, especially for tasks that don’t require extreme precision. It’s always worth trying them out to see how they fit your specific needs. Thanks for your interest and great question!
That RTX 5090 really seems worth it for handling those big models! I wonder how much difference the quantization makes in practical use cases though. Your insights on performance variability between models are super interesting.
Absolutely, quantization can make a noticeable difference, especially when you’re resource-constrained. In practice, it often allows larger models to fit into smaller memory footprints without sacrificing too much accuracy. Your observation about performance variability is spot-on; it’s one of the most fascinating aspects to explore. Thanks for your insightful comment—it really helps spark deeper discussions!
I had no idea the RTX 5090 made such a huge difference in performance! It’s fascinating how much smoother the models run compared to setups with less VRAM. Have you noticed any specific tasks where the local deployment really shines?
Absolutely! The RTX 5090’s massive VRAM really shines in complex multi-modal tasks like training large language models with extensive datasets or running heavy inference workloads. I’ve also seen it make a big difference in maintaining stable performance during long training sessions without bottlenecks. Thanks for your insightful comment—these discussions always help highlight key takeaways!
I had no idea the RTX 5090 could handle these models so smoothly despite their size—definitely makes me reconsider local deployment. It’s wild how much faster fine-tuning feels compared to cloud-based options, but managing all that power must still require some serious technical know-how. Your insights on quantization really highlighted the trade-offs involved—it’s not just about raw performance.
I didn’t realize how much VRAM the deepseek r1 distilled version actually needs! It’s fascinating that even with such a beastly GPU, there are still moments where it feels bottlenecked. Your insights on balancing batch sizes versus generation speed were really eye-opening for someone considering this kind of setup.